Diffusion for Multi-Embodiment Grasping
Roman Freiberg, Alexander Qualmann, Ngo Anh Vien, Gerhard Neumann
IEEE Robotics and Automation Letters (2025)
Abstract
Grasping is a fundamental skill in robotics with diverse applications. However, current approaches for predicting valid grasps are often tailored to specific grippers, limiting their applicability when gripper designs change. To address this limitation, we explore the transfer of grasping strategies between various gripper designs, enabling the use of data from diverse sources. We present an approach based on equivariant diffusion that facilitates gripper-agnostic encoding of scenes containing graspable objects and gripper-aware decoding of grasp poses by integrating gripper geometry into the model. We also develop a dataset generation framework that produces cluttered scenes with variable-sized object heaps, improving the training of grasp synthesis methods. Experimental evaluation on diverse object datasets demonstrates the generalizability of our approach across gripper architectures, ranging from simple parallel-jaw grippers to humanoid hands, outperforming both single-gripper and multi-gripper state-of-the-art methods.
Method Overview
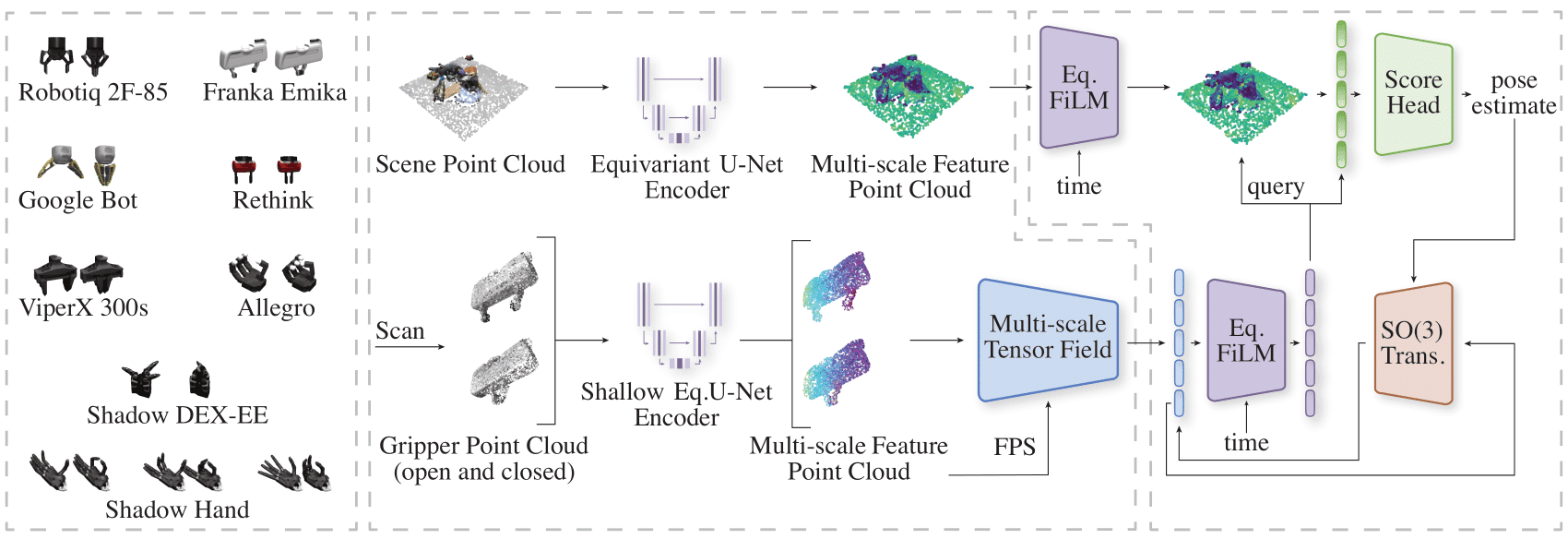
(a) Overview of open and closed gripper configurations. (b) Scene and gripper point clouds are encoded through an equivariant U‑Net, producing multi‑scale hierarchical equivariant descriptor field (EDF) features. A multi‑scale tensor field then computes query positions and EDF features using the Farthest Point Sampling (FPS) algorithm. (c) The featurized point clouds and query tokens are time‑conditioned via an equivariant FiLM layer. The tokens query features in the scene’s hierarchical point cloud, which the score head uses to predict a diffusion‑based pose estimate. The resulting pose estimate is applied as an equivariant transform to the EDF features of the query tokens.
Demo Video
Cite Us
@article{freiberg2025diffusion,
title={Diffusion for Multi-Embodiment Grasping},
author={Freiberg, Roman
and Qualmann, Alexander
and Vien, Ngo Anh
and Neumann, Gerhard},
journal={IEEE Robotics and Automation Letters},
year={2025},
publisher={IEEE}
}